Why Data Science Matters
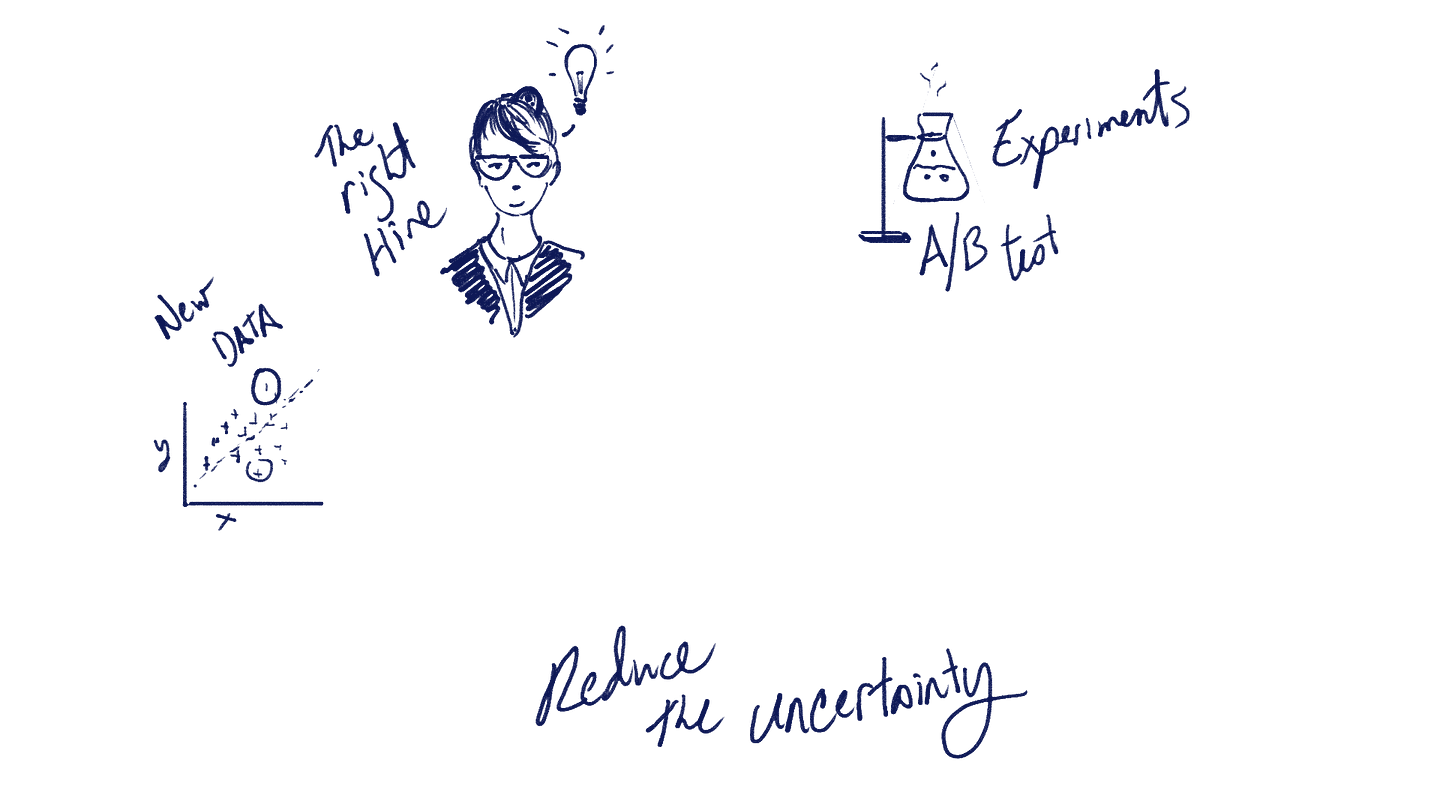
In the first post of our series on building data-informed companies, we shared the most important ingredients needed to build a data-informed company: singular focus on impact and building a data-informed culture. In this second post, we will focus on why data science matters and discuss the future of data science in the context of building products.
What, exactly, is data science? Data science is a scientific and truth-seeking discipline that uses data to extract knowledge and insights. Data science is one of the fastest growing functions and is already providing tremendous value across every industry and area of study. Nevertheless, data science is still in its infancy and like any developing field, it is often tempting to put boundaries around its definition. Rather than categorizing what does or does not count as data science or arguing about why we should be data-informed but not data-driven, we believe it is most important to leave room for the discipline to evolve organically.
WHY DOES DATA SCIENCEÂ MATTER?
Starting a tech company, building a good product, and gaining traction have become easier thanks to improved connectivity, declining cloud storage and computing costs, and the accessibility of distribution platforms for reaching target audiences. As a result, the time it takes a product to reach 100 million monthly active users has shortened dramatically, and continues to shrink today. For example, it took about 100 months for iTunes to reach 100 million monthly active users in 2003 and mere days for Pokemon Go to do the same. The chart below has some more good examples, starting with the telephone.
The combination of more products built, more Internet-connected devices bought, and increased time spent online has caused a spike in the volume of user interaction data. There has been a tremendous interest in mining this data and deriving key insights to help build great products. A company’s ability to compete is now measured by how successfully it applies analytics to vast, unstructured data sets across disparate sources to drive product innovation. Therefore, data scientists are in high demand, and that a team of smart data scientists can make or break a product.
This increasing interest in mining data for insights has led product teams to use data to focus on four specific outcomes.
- Evaluate the health of the business: One of the key outcomes of product analysis is to evaluate the health of a product or a business. Once we have defined product success by the means of a goal and a metric, the next step is to monitor the metric to ensure that we are on track to hitting the goal. Tactically, analysts work on identifying outliers, understanding the drivers of changes in metrics, building dashboards / reports / visualizations etc.
- Ship the right products and features: Another very important role of analytics is to ensure that the right products and features get built. Many companies run numerous experiments and ship products after evaluating the results of these experiments. Typically data scientists help design experiments, identify data-informed hypotheses on phenomena, and guide product team on constant optimization of the product through the data insights.
- Forecast outcomes and Power production systems — Another role of data scientists is to build prototypes/models and power production systems using AI/ML. These data scientists train machine learning models of a phenomenon in order to forecast future expectations and trends.
- Set roadmap and strategy for the product: Deeper exploration and analysis of the user journey and phenomena generate actionable insights that ultimately result in setting roadmap and strategy for the product. Data-driven roadmap and strategy is one of the most important outputs of a world class product analytics team.
These four outcomes have specifically led to two different types of data scientists in the industry — product analysts and algorithm developers.
WHAT DO DATA SCIENTISTS DO?
The title of data scientist encompasses multiple roles that vary significantly across companies and industries. That said, generally speaking there are two main camps of data scientists:
- Product analysts, whose role is to deliver data-informed stories that advocate for a change in product or strategy. E.g., Our SMS notification delivery system is broken in India. As a result, we need to focus on improving SMS notifications in India, which will help reignite growth.
- Algorithm developers, whose role is to incorporate data-driven features into products (e.g., optimizing recommendations or search results). E.g. The fraud levels in Indonesia has increased. Build a new model that focuses on the recent fraud trend in Indonesia.
Product analysts focus on setting goals and informing product roadmaps and strategies. They help improve products by evaluating and understanding their health and providing product decisions (largely via experimentation). The general deliverable from product analysts is a document to the product team narrating quantifiable issues, identified opportunities, and data-based recommendations and solutions.
An algorithm developer’s primary job is to leverage data to improve product performance in pursuit of a specific end goal, typically forecasting outcomes or building production systems. Algorithm developers generally use machine learning and other complex algorithmic techniques to make predictions based on inputs from vast quantities of data. In general, algorithm developers prototype proposed solutions and work closely with engineering teams to implement them in production. The deliverable from algorithm developers is prototyped code and documentation that get provided to the engineering team.
While both types of data scientists require an analytical outlook, quantitative skills, and the ability to prioritize, it is rare to find a person who can fit both roles. Algorithm developers require more sophisticated technical knowledge, such as machine learning and artificial intelligence, and a level of software engineering skills closer to those of engineers. Product analysts are primarily problem solvers who are differentiated based on their business, product, and ability to communicate effectively to a wide variety of stakeholders.
While only some organizations require algorithm developers, all companies, especially those with a significant user base, benefit from product analysts who can help navigate product, competitive, and other strategic challenges. Later in this series of blog posts, we will provide additional guidance on hiring, training, coaching and managing product analysts so they can contribute at the highest levels.
So, do companies need to hire algorithm developers and be more data-driven or should they hire product analysts and be more data-informed? Outcomes are purely data-driven when data is the only signal required to make a decision. In contrast, in data-informed decisions, data is a strong input but not the only input. Generally speaking, Product Analysts are data-informed and algorithm developers are data-driven.
EVOLUTION OF DATAÂ SCIENCE
Imagine a world in which a machine knows everything about you and can shop for you without even explicitly asking for it; knows the food you like and cooks for you; knows your choices and can make decisions for you and knows what is good for you and plans your life. This world is distant into the future and requires Artificial Intelligence to take over much of our lives. For us to make progress toward this dream, we need to become even more data-driven.
In a perfect world with perfect information and a complete understanding of all the drivers of your system and how they interact with each other, the two approaches would converge. In order to build a perfect model, the phenomenon under study needs to be completely understood; the relationship between the data and the phenomenon can be described by a perfect model (and associated rich feature set). In order to evolve to this level of perfection and also make progress in the interim, the world will need to continue to make progress on data-informed decision-making. i.e., we need to continue augmenting our decision making by other subjective measures that cannot easily be fully quantified yet. As we begin to have a deeper understanding of relationships between objects, more and more processes will get automated away and the future will be more data-driven than data-informed. However, data-informed decision making will continue to be extremely important for the next few decades and data-driven decision making will only improve with advancements from people who are data-informed.
It is most illustrative to understand the differences between data-informed decision making and data-driven decision making by means of examples.
- Setting goals. Good goals are measurable and quantifiable. Being able to identify and track goals will become increasingly data-driven. For example, Facebook’s tracking of its active users maybe completely automated. However, setting the right quarterly and annual goals for active users and revenue maybe only partly automated and will continue to be data-informed.
- Defining a roadmap and strategy. Establishing a roadmap and strategy is not quantitative and hence requires data-informed approaches. For example, by using data, a roadmap can be developed for increasing daily active usage by focusing on SMS notification. A good roadmap considers the relevant goals, the drivers of these goals, the levers that the product team has, and all of the courses of action that can be taken. Much of this is qualitative, so the process of building a roadmap and defining strategy is primarily data-informed.
- Forecasting outcomes. Forecasting outcomes is mostly data-driven. For example, figuring out whether or not to show a story to a user would require understanding multiple factors, including the probability of a user clicking or reading that story. Companies typically develop models, which are iterated on continuously, to forecast this specific outcome.
- Powering production systems. For companies like PayPal to identify fraudulent activity of any transaction, it is prohibitively expensive to do this manually for all transactions. As a result, they largely rely on machine learning to power their production systems and automate the calculation of the probability of a transaction being bad. Much of the decision-making that follows the evaluation of the probability is also automated. However, in areas where there are lower levels of confidence in the probability evaluations, the decision process could be data-informed.
TAKEAWAYS
- Improving products and monetizing through data has become a competitive advantage in recent years. A strong and well-organized data organization is a strong differentiator.
- Data scientists are driving key product decisions across companies and building next-generation algorithms to improve decision making.
- The world will continue to become increasingly data-driven, but data-informed decision making will remain relevant.
This work is a product of Sequoia Capital’s Data Science team. Chandra Narayanan, Hem Wadhar and Ahry Jeon wrote this post. See the full data science series here.Â